Label Images for Object Detection: A Comprehensive Guide
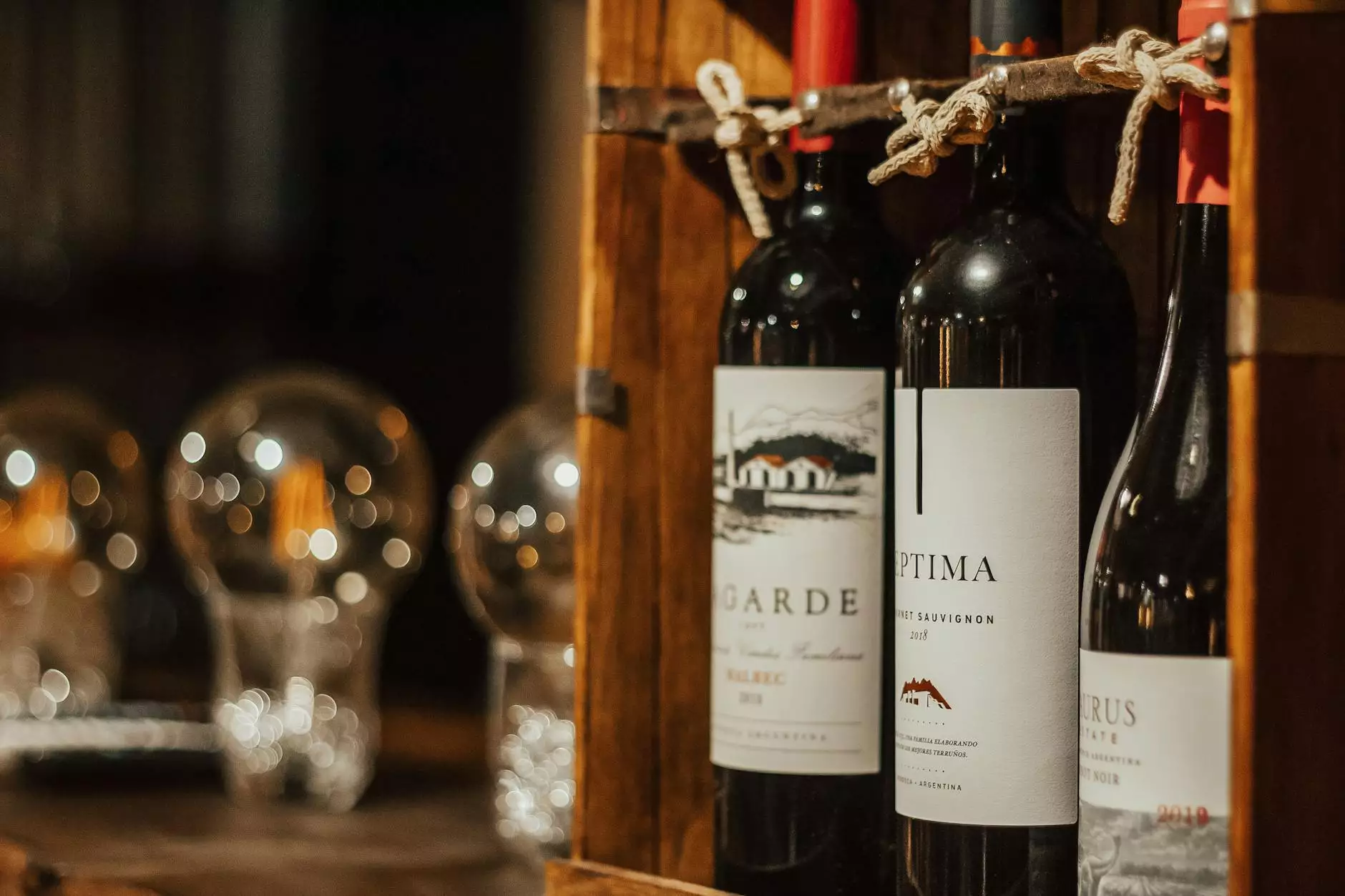
The field of object detection has seen remarkable advances due to technological innovations and increased demand for automated systems. With applications spanning from self-driving cars to video surveillance, the importance of accurate labeling and data annotation cannot be overstated. In this article, we delve into the intricacies of how to label images for object detection, highlighting effective strategies and tools available, particularly focusing on the exceptional offerings from Keylabs.ai.
Understanding Object Detection
Object detection involves identifying instances of objects within images and videos, and classifying them into predefined categories. The development of deep learning algorithms has revolutionized this field by automating feature extraction and classification tasks. However, the effectiveness of these algorithms hinges significantly on the quality of the labeled training data.
The Importance of Image Labeling
- Training Data Quality: High-quality labeled images improve the accuracy of object detection algorithms.
- Data Diversity: A diverse set of labeled images ensures the model generalizes well across different scenarios.
- Efficiency: Proper labeling can minimize the amount of data needed for training while maximizing performance.
Key Features of Effective Image Labeling
When it comes to labeling images for object detection, several characteristics contribute to effective results:
1. Precision in Labeling
Each image needs to be meticulously labeled to avoid misinterpretation by the model. This involves not only recognizing the objects but also detailing their boundaries accurately using annotations. The most common formats include:
- Bounding Boxes: Rectangles drawn around objects to indicate their positions.
- Polygons: More precise shapes for complex objects.
- Segmentation Masks: Pixel-level labeling for high accuracy in object representation.
2. Consistency Across Labels
It is vital to maintain consistency in labeling practices. This means that the same type of object should always be labeled in the same manner across the dataset. Using well-defined labeling guidelines can help achieve this consistency.
3. Proper Tool Selection
Choosing an effective data annotation tool can significantly enhance productivity and quality in image labeling. Tools should be user-friendly, allow for collaboration among team members, and provide various annotation options. Keylabs.ai stands out in this regard, offering an intuitive platform with a range of functionalities.
Using Keylabs.ai for Image Labeling
At Keylabs.ai, the focus is on providing top-tier data annotation solutions that streamline the process of labeling images for object detection. Let's explore how you can leverage their platform effectively:
Features of Keylabs.ai
- User-Friendly Interface: Keylabs.ai provides an intuitive dashboard, making it easy for users to navigate and execute tasks efficiently.
- Collaborative Working Environment: The platform allows teams to collaborate seamlessly, improving efficiency in the annotation workflow.
- Multiple Annotation Types: Users can create bounding boxes, polygons, and segmentation masks effortlessly, catering to various project needs.
- Quality Control Mechanisms: Keylabs.ai integrates quality checks to ensure that the labeled data meets high standards before it is used in training machine learning models.
A Step-by-Step Guide to Labeling Images for Object Detection
Whether you are working on a small project or a large dataset, the steps to label images for object detection remain broadly the same. Here’s a detailed breakdown:
Step 1: Define the Annotation Guidelines
Before you begin, create clear and concise guidelines for how you'll label images. This should include definitions of classes, labeling formats to be used, and examples of proper labeling.
Step 2: Choose the Right Tools
Select a data annotation tool that fits your project requirements. If you are looking for advanced features and user support, Keylabs.ai is a solid choice.
Step 3: Upload Your Dataset
Upload the images you want to label to your selected platform. Organize your images into logical folders to simplify the labeling process.
Step 4: Begin Labeling
Start the annotation process by selecting an image and applying your predefined labels. Focus on accuracy and consistency, and consider involving team members to enhance productivity.
Step 5: Review and Quality Assurance
After labeling the images, conduct a thorough review to ensure all annotations meet the established guidelines. Implement quality control measures to validate the accuracy of your labeled data.
Step 6: Export Your Labeled Data
Once satisfied with the quality of annotations, export your labeled dataset in the desired format. Ensure compatibility with your machine learning models.
Common Challenges in Image Labeling for Object Detection
Labeling images for object detection can be a complex process fraught with difficulties. Some common challenges include:
1. High Volume of Data
A large dataset can be overwhelming. It's essential to strategize and possibly use automation tools to assist with the labeling process.
2. Ambiguity in Object Categories
Sometimes, boundaries between different categories can be unclear. Providing comprehensive guidelines and examples can mitigate this issue.
3. Errors in Annotated Data
Even skilled annotators can make mistakes. Regular quality assurance processes can help catch and rectify these errors before the data is used for training.
Future Trends in Image Labeling Technologies
The field of data annotation is evolving quickly. Upcoming trends you should be aware of include:
1. Automation and AI-assisted Labeling
As machine learning continues to advance, we anticipate significant developments in automated image labeling technologies, which will help to significantly reduce the time and effort required.
2. Improved Collaborative Tools
The future will likely see an increase in platforms that facilitate real-time collaboration among teams working remotely on labeling tasks.
3. Enhanced Quality Control Systems
AI-driven quality assurance tools will improve the consistency and accuracy of annotations, helping to streamline workflows and optimize results.
Conclusion
In the growing field of object detection, the ability to label images for object detection accurately is paramount for the success of machine learning applications. By employing the right strategies and leveraging advanced tools like those offered by Keylabs.ai, you can ensure high-quality data annotation that ultimately enhances the performance of your models. Remember, the quality of your training data determines the effectiveness of your object detection algorithms. Therefore, investing time and resources into masterful image labeling is an investment in your project's success.