Understanding Semantic Segmentation in Video: A Comprehensive Guide
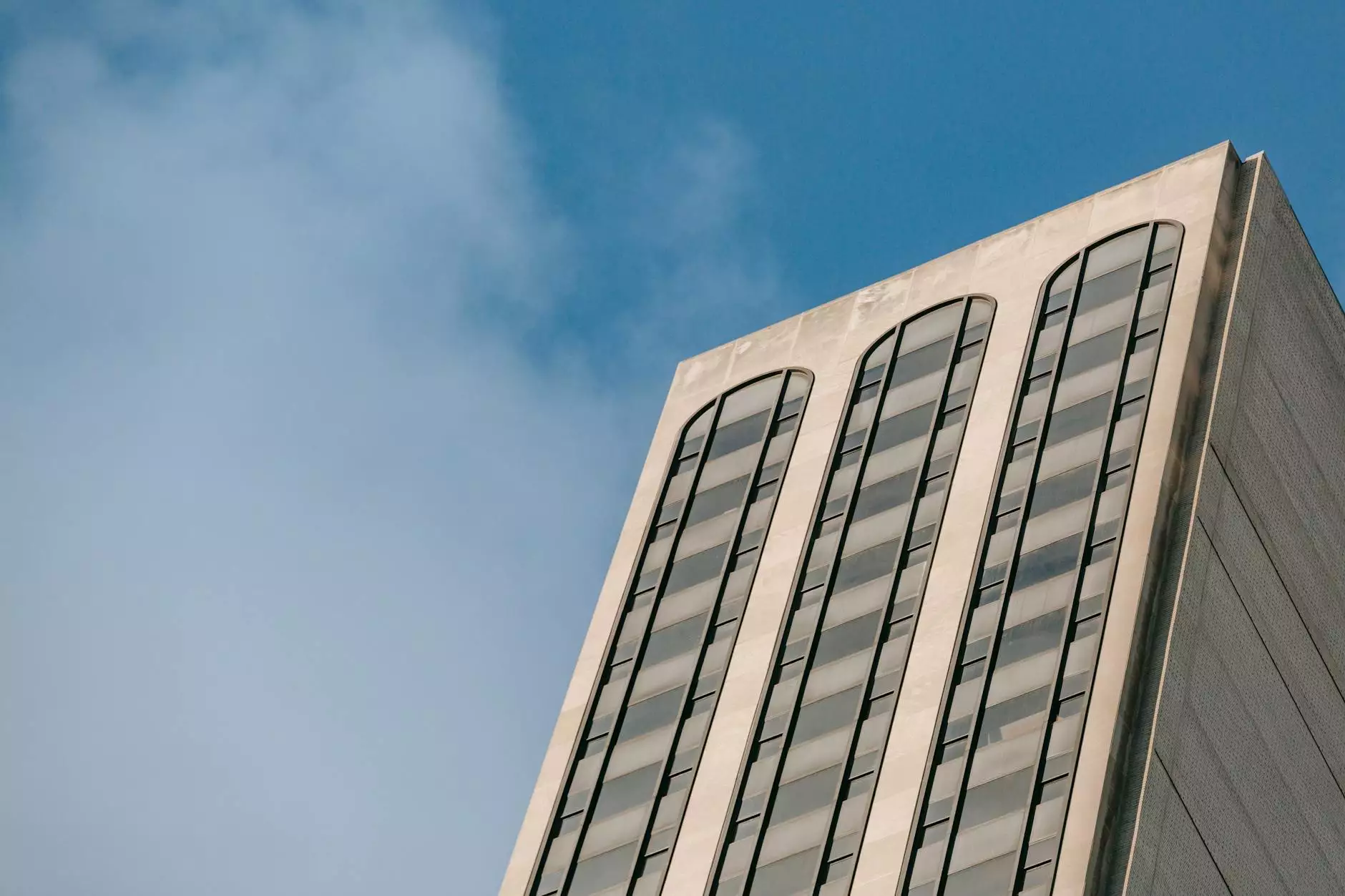
In an era dominated by data and machine learning, the semantic segmentation video has emerged as a pivotal technology for accurately interpreting visual data. With applications spanning across various industries such as autonomous vehicles, healthcare, and security, the ability to segment objects within a video frame not only enhances visual understanding but also facilitates advanced analytics. At Keylabs.ai, we delve into the intricacies of this technique and provide robust solutions through our state-of-the-art data annotation tools and data annotation platform.
What is Semantic Segmentation?
Semantic segmentation refers to the process of partitioning an image or video into regions based on the semantic objects present. Unlike traditional segmentation, which focuses on merely identifying outlines, semantic segmentation involves classifying each pixel within the video frames into specific categories, allowing machines to understand the content in a more nuanced manner.
The Importance of Semantic Segmentation in Video Analysis
The evolution of technology has led to a growing demand for semantic understanding of visual content. The applications of semantic segmentation video extend into numerous sectors:
- Autonomous Vehicles: Segmentation helps in recognizing pedestrians, vehicles, and road signs, ensuring safer navigation.
- Healthcare: Applications include automated analysis of medical imaging, facilitating precise diagnostic procedures.
- Video Surveillance: Enhances the monitoring capabilities by identifying specific objects or individuals in the frame.
- Industrial Automation: In manufacturing, it is used for quality control and monitoring production lines.
How Does Semantic Segmentation Work?
Semantic segmentation relies heavily on deep learning algorithms, especially those employing Convolutional Neural Networks (CNNs). Below are the fundamental steps involved:
1. Data Collection
The first step is gathering a robust dataset containing diverse video frames. This data is crucial for training machines to recognize various categories of objects accurately.
2. Data Annotation
Next comes data annotation, where human expertise is essential. Each video frame is manually annotated to label pixel-wise categories, making it possible for the model to learn from the training data. Platforms like Keylabs.ai offer advanced tools for efficient data annotation, ensuring precision and quality.
3. Model Training
The annotated dataset is then utilized to train models. This step involves feeding the training data into CNNs to learn the relationships between pixels and their respective labels.
4. Testing and Validation
Once the model is trained, it undergoes rigorous testing with unseen data to evaluate its accuracy and performance. Continuous iterations may be necessary to refine the model further.
5. Implementation
Finally, once validated, the model is deployed to perform real-time semantic segmentation on video streams, providing valuable insights and data processing capabilities.
Challenges in Semantic Segmentation Video
Despite its impressive capabilities, semantic segmentation in video still faces several challenges:
- Extensive Data Requirements: High-quality, annotated training datasets are often scarce and expensive to produce, making it challenging to achieve high accuracy.
- Occlusion and Ambiguity: Objects can occlude each other in video streams, making it difficult for models to segment overlapping objects properly.
- Dynamic Environments: Changes in lighting, weather conditions, and movement can affect segmentation performance in real-time applications.
- Computational Complexity: Processing video streams in real-time requires significant computational resources, which can be a barrier for deployment.
The Role of Data Annotation Tools
Addressing the challenges of semantic segmentation video effectively hinges on the right data annotation tools. At Keylabs.ai, our platform is built to meet the diverse needs of businesses engaged in data-intensive projects. Here are some features that set our platform apart:
- User-Friendly Interface: Our intuitive interface allows for easy navigation, making the annotation process more efficient.
- Automation Features: Advanced machine learning-driven automation tools can expedite the annotation process while maintaining high accuracy.
- Collaboration Tools: Facilitates teamwork by allowing multiple users to work on the same project seamlessly.
- Quality Assurance: Built-in QA tools ensure that annotated data meets the highest standards before it's used for training.
Best Practices for Effective Semantic Segmentation Video
To maximize the efficacy of semantic segmentation, consider the following best practices:
1. Invest in Quality Data
The success of your semantic segmentation model is heavily reliant on the quality of your training data. Invest time and resources in creating a well-annotated dataset that represents the variety of scenarios your model will encounter in the real world.
2. Leverage Advanced Annotation Tools
Utilize powerful tools like those offered by Keylabs.ai to annotate your data efficiently and accurately. These tools can save time and reduce human error.
3. Continuous Training and Adjustment
Machine learning is not a one-time effort; models should be continuously retrained and adjusted to improve performance as new data becomes available.
4. Monitor Performance Regularly
Regular performance evaluation is crucial. Use metrics such as Intersection Over Union (IoU), precision, and recall to assess and fine-tune your models.
5. Adapt to Changes
Stay adaptable to new technologies and methodologies in the field of semantic segmentation. Innovations in deep learning and data annotations can significantly enhance your outcomes.
Future Trends in Semantic Segmentation Video
The field of semantic segmentation is continually evolving, and keeping an eye on emerging trends can prepare businesses to leverage new opportunities. Notable trends include:
- Integration with Augmented Reality (AR): Enhancements in AR applications will depend heavily on effective semantic segmentation capabilities for real-time interaction.
- Increased Use of Synthetic Data: The potential of synthetic data generation will reduce costs and improve model training efficiency.
- Real-time Processing Advances: Ongoing research to optimize model architectures will facilitate faster and more efficient real-time video processing.
- Cross-Domain Adaptation: Techniques allowing models trained on one domain to perform well in another domain will be highly sought after.
Conclusion
Semantic segmentation video is not merely a technological advancement; it represents a substantial leap toward understanding and processing visual information with precision. By harnessing robust data annotation tools and platforms, businesses can unlock the potential of their data, leading to enhanced decision-making and operational efficiencies. At Keylabs.ai, we are committed to providing tailored solutions for your data annotation needs, ensuring that your organization stays at the forefront of the AI revolution.
As you embark on your journey toward integrating semantic segmentation video into your strategies, remember that the quality and precision of your data annotation processes will ultimately dictate your success. Embrace the future of data with confidence.